Configuring experiments
The configuration of experiments consists of selecting the target and the features that the model will use to predict the target. You can also configure a number of optional settings.
To support you in the selection of a target, the historical dataset is analyzed and summary statistics are displayed about each column in the dataset. Several automatic preprocessing steps are applied to the dataset to make sure that only suitable data is included. For more details on the data preprocessing, see Automatic data preparation and transformation.
After running v1, you can create new experiment versions if needed to further refine the model training. For more information, see Refining models.
Requirements and permissions
To learn more about the user requirements for working with ML experiments, see Working with experiments.
Views
The default view is the schema view, where each column in your dataset is represented by a row in the schema with information and statistics. To get more information and sample data for each column you also have the data view. Click and
to change between the views.
A preview of the dataset shown in schema view
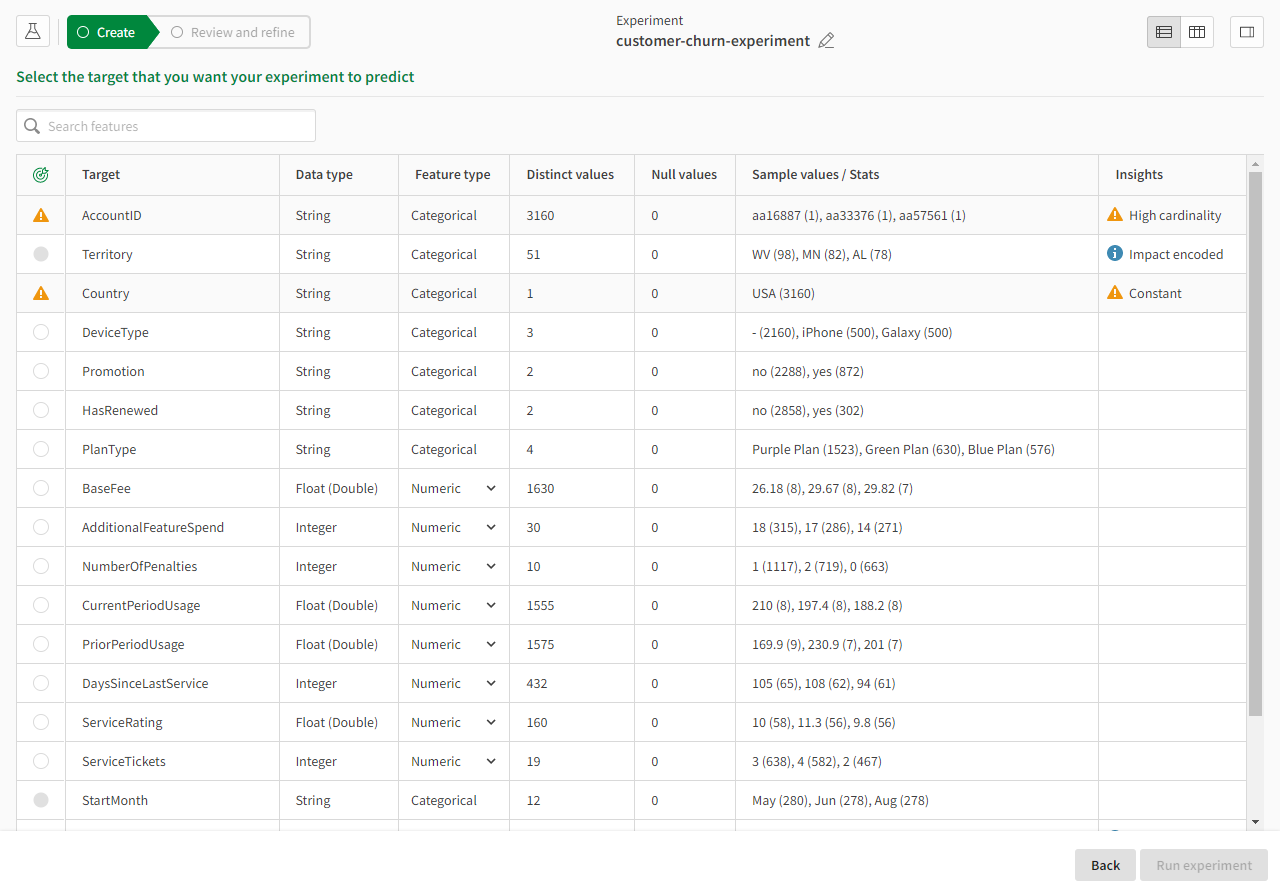
A preview of the dataset shown in data view
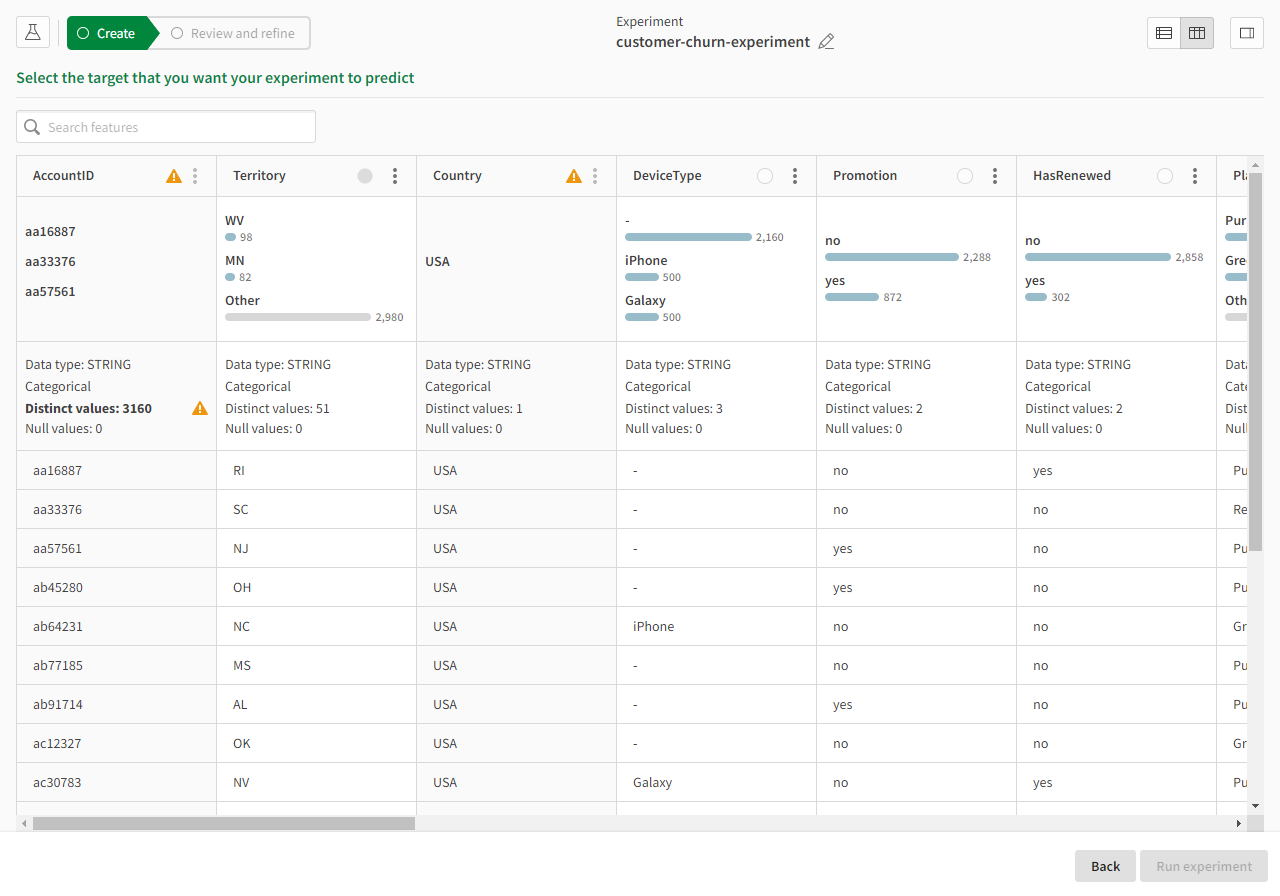
Click to open or close the Experiment configuration side pane. Here you find information about your experiment and the current configuration.
The side pane shows the experiment configuration for the current version
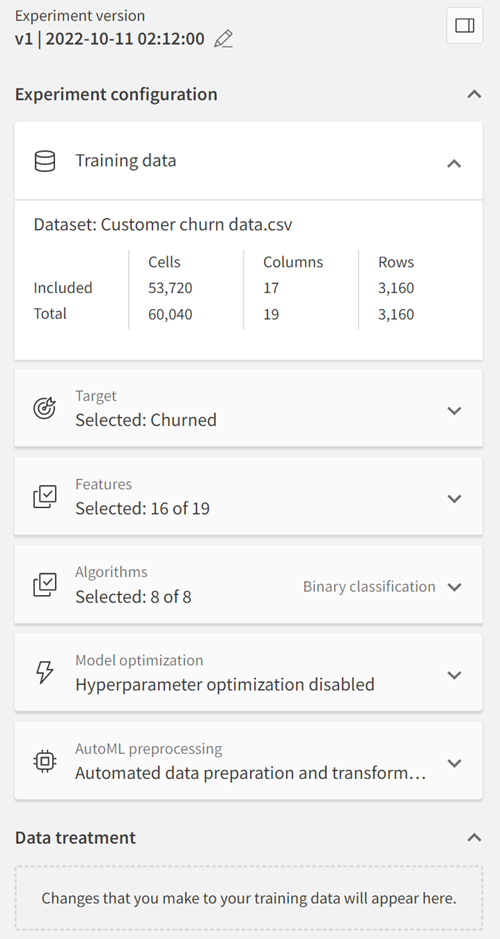
Selecting a target
The target column contains the values that you want the machine learning model to predict. You can change the target column until you start the first training. After that, it is locked for editing.
Do the following:
-
Hover over the column and click the
icon that appears.
The target column is now indicated by
and the other available columns are automatically selected as features.
Selecting the target

When the target is selected, you can start running the first version of the experiment. Read more in Training experiments. You can do additional configuration at this point—described below—or adjust the configuration after you have reviewed the training results.
Explanations of how your data is being interpreted and processed are shown as you navigate experiment training. For more information, see Common insights found in training data.
Determining the type of model created
The column you select as the target determines the type of model your experiment creates. This, in turn, plays a part in determining which algorithms are used to train the model. Certain columns in your dataset may not be selectable as a target for your experiment, or may have specific processing applied to them.
The model types are:
-
Binary classification model
-
Multiclass classification model
-
Regression model
The table below summarizes the factors in your target that determine the type of model used.
Model type | Number of distinct values in column | Feature type required | Additional information |
---|---|---|---|
Binary classification | 2 | Any | - |
Multiclass classification | 3-10 | Any | A column with more than 10 distinct, non-numeric classes is not selectable as the target. |
Regression | More than 10 | Numeric | - |
Selecting feature columns
With the target set, you can choose which of the other available columns to include in the training of the model. Exclude any features that you don't want to be part of the model. Note that the column will stay in the dataset but will not be used by the training algorithm.
At the top of the Experiment configuration pane, you can see the number of cells in your dataset. If the number exceeds your dataset limit, you can exclude features to get below the limit.
You can select the feature columns in various ways:
-
Manually clear the checkboxes for the features you don't want to include.
-
Deselect Include all available features and then select only the ones you want to include.
-
Make a search and exclude or include all features in your filtered search result.
-
After you have run the first version of the experiment, you can define the Number of top features to include.
Features section in the experiment configuration
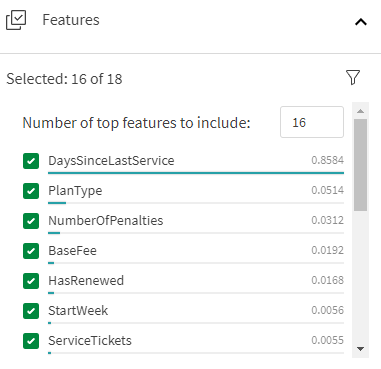
When you select features, they are automatically assigned a feature type. The possible feature types are:
-
Categorical
-
Numeric
-
Date
-
Free text
The feature type is assigned based on the data contained in the feature column. If a feature meets certain criteria, it might be staged to become the basis for auto-engineered features. If desired, you can change whether the feature is used for automatic feature engineering. For full details about automatic feature engineering, see Automatic feature engineering.
Certain columns in your dataset may not be selectable as features for your experiment, or may have specific processing applied to them. Explanations of how your data is being interpreted and processed are shown as you navigate experiment training. For more information, see Common insights found in training data.
Selecting algorithms
All available algorithms are included by default and you can exclude any algorithms that you don't want to use. Normally, you would do this as part of the model refinement when you have seen the first training results. Read more in Refining models.
Algorithms section in the experiment configuration
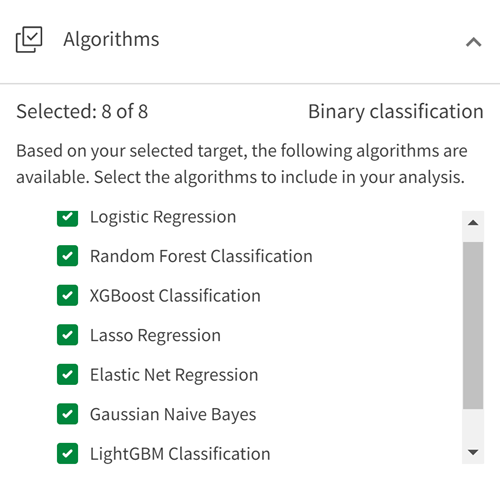
Changing feature types
When a dataset is loaded, the columns are treated as categorical, numeric, date, or free text based on the data type and other characteristics. In some cases, you might want to change this setting.
For example, if the days of the week are represented by the numbers 1-7, each number represents a categorical value. By default, it is treated as a continuous ranked numeric value, so you would need to manually change the configuration to treat it as categorical.
When a column is identified as containing date and time information, it is used as the basis for new generated auto-engineered features. When this happens, the original column (the parent feature) is treated as having the date feature type. You can change the parent feature from a date feature type to a categorical feature type. However, if you do this, you can no longer use its auto-engineered features in experiment training.
Do the following:
-
In
Schema view, locate the feature.
- In the Feature type column for this feature, click
.
-
In the Feature type column, click
.
-
Select a value in the list.
You can see all columns that have a changed feature type on the Experiment configuration pane under Data treatment.
Changing dataset
You can change the training dataset before you run the first experiment version, as well as after running any version.
If you change the dataset before running the first version, you will lose any configuration that you have done prior to changing the dataset.
Do the following:
-
On the Experiment configuration pane under Training data, click Change dataset.
-
Select a new dataset.
For more information about changing and refreshing the dataset during model refinement (after running an experiment version), see Changing and refreshing the dataset.
Configuring hyperparameter optimization
You can optimize the model using hyperparameter optimization. Note that this is an advanced option that could increase the training time significantly. For more information, see Hyperparameter optimization.
Model optimization section in the experiment configuration
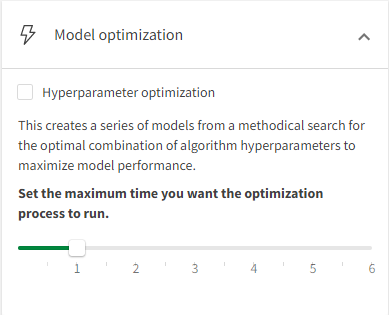
Do the following:
-
On the Experiment configuration pane, expand the Model optimization section.
-
Select the Hyperparameter optimization checkbox.
-
Optionally, set a time limit for your optimization. The default time limit is one hour.
Common insights found in training data
Depending on the quality of your dataset, there might be limitations on how you can use specific parts of the data in your experiment configuration. The Insights column in schema view is helpful in identifying particular characteristics of data fields and how they will be processed by machine learning algorithms.
The following table shows possible insights that may be displayed in the schema:
Insight | Meaning | Impact on configuration |
---|---|---|
Constant | The column has the same value for all rows. | The column can't be used as a target or included feature. |
One-hot encoded | The feature type is categorical and the column has less than 14 unique values. | No effect on configuration. |
Impact encoded | The feature type is categorical and the column has 14 or more unique values. | No effect on configuration. |
High cardinality | The column has too many unique values, and can negatively affect model performance if used as a feature. | The column can't be used as a target. It will be excluded automatically as a feature, but can still be included if needed. |
Sparse data | The column has too many null values. | The column can't be used as a target or included feature. |
Underrepresented class | The column has a class with less than 10 rows. | The column can't be used as a target, but can be included as a feature. |
<number of> auto-engineered features | The column is the parent feature that can be used to generate auto-engineered features. | If this parent feature is interpreted as a date feature, it is automatically removed from the configuration. It is recommended that you instead use the auto-engineered date features that can be generated from it. It is possible to override this setting and include the feature rather than the auto-engineered features. |
Auto-engineered feature | The column is an auto-engineered feature which can, or has been, generated from a parent date feature. It did not appear in the original dataset. | You can remove one or multiple of these auto-engineered features during experiment training. If you switch the feature type of the parent feature to categorical, all auto-engineered features are removed. |
Could not process as date | The column possibly includes date and time information, but could not be used to create auto-engineered date features. | The feature is dropped from the configuration. If auto-engineered features were previously generated from this parent feature, they are removed from future experiment versions. You can still use the feature in the experiment, but you must switch its feature type to categorical. |
Possible free text | The column could possibly be available for use as a free text feature. | The free text feature type is assigned to the column. You must run an experiment version to confirm whether the feature can be processed as free text. |
Free text | The column has been confirmed as containing free text. It can be processed as free text. | No additional configurations are required for the feature. |
Could not process as free text | Upon further analysis, the column cannot be processed as free text. | You need to deselect the feature from the configuration for the next experiment version. If the feature does not have high cardinality, you can alternatively change the feature type to categorical. |