Modeling the accident-prone areas in a city
This scenario applies only to Talend products with Big Data.
For more technologies supported by Talend, see Talend components.
In this scenario, the tKMeansModel component is used to analyze a set of sample geographical data about the destination of ambulances in a city in order to model the accident-prone areas.
A model like this can be employed to help determine the optimal locations for building hospitals.
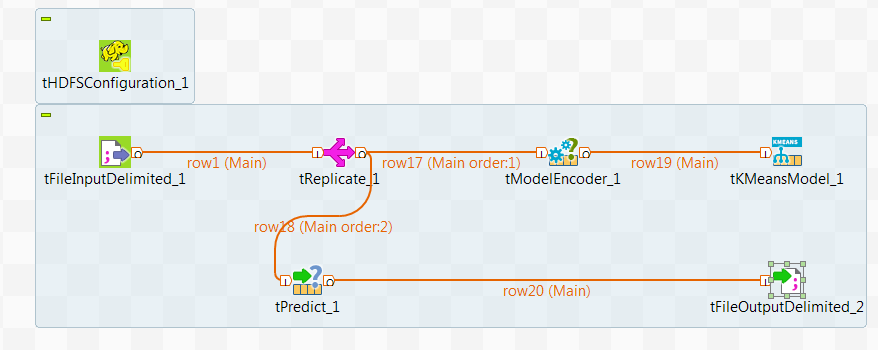
You can download this sample data from here. It consists of pairs of latitudes and longitudes.
The sample data was randomly and automatically generated for demonstration purposes only and in any case it does not reflect the situation of these areas in the real world.
-
The Spark version to be used is 1.4 onwards.
-
The sample data is stored in your Hadoop file system and you have proper rights and permissions to at least read it.
-
Your Hadoop cluster is properly installed and is running.
-
tFileInputDelimited: it loads the sample data into the data flow of the Job.
-
tReplicate: it replicates the sample data and caches the replication.
-
tKMeansModel: it analyzes the data to train the model and writes the model to HDFS.
-
tModelEncoder: it pre-process the data to prepare proper feature vectors to be used by tKMeansModel.
-
tPredict: it applies the KMeans model on the replication of the sample data. In the real-world practice, this data should be a set of reference data to test the model accuracy.
-
tFileOutputDelimited: it writes the result of the prediction to HDFS.
-
tHDFSConfiguration: this component is used by Spark to connect to the HDFS system where the jar files dependent on the Job are transferred.
In the Spark Configuration tab in the Run view, define the connection to a given Spark cluster for the whole Job. In addition, since the Job expects its dependent jar files for execution, you must specify the directory in the file system to which these jar files are transferred so that Spark can access these files:-
Yarn mode (Yarn client or Yarn cluster):
-
When using Google Dataproc, specify a bucket in the Google Storage staging bucket field in the Spark configuration tab.
-
When using HDInsight, specify the blob to be used for Job deployment in the Windows Azure Storage configuration area in the Spark configuration tab.
- When using Altus, specify the S3 bucket or the Azure Data Lake Storage for Job deployment in the Spark configuration tab.
- When using Qubole, add a tS3Configuration to your Job to write your actual business data in the S3 system with Qubole. Without tS3Configuration, this business data is written in the Qubole HDFS system and destroyed once you shut down your cluster.
-
When using on-premises distributions, use the configuration component corresponding to the file system your cluster is using. Typically, this system is HDFS and so use tHDFSConfiguration.
-
-
Standalone mode: use the configuration component corresponding to the file system your cluster is using, such as tHDFSConfiguration Apache Spark Batch or tS3Configuration Apache Spark Batch.
If you are using Databricks without any configuration component present in your Job, your business data is written directly in DBFS (Databricks Filesystem).
-